Introduction to GenericModel
Overview:
The GenericModel
module in ADS provides different ways of serializing a
trained model. This example demonstrates how to utilize the
GenericModel
module to prepare model artifacts, and save models to the
model catalog then deploy any unsupported model framework.
A model artifact includes the model, metadata about the model, input and output schema, and a script to load the model and make predictions. You can share model artifacts among data scientists, tracked for provenance, reproduced, and deployed.
Initialize
GenericModel()
initiates a generic model instance. It accepts the following variables:
estimator: (Callable)
. Trained model.artifact_dir: str
. Artifact directory to store the files needed for deployment.properties: (ModelProperties, optional)
. Defaults toNone
. ModelProperties object required to save and deploy model.auth :(Dict, optional)
. Defaults toNone
. The default authentication is set using theads.set_auth
API. If you need to override the default, useads.common.auth.api_keys
orads.common.auth.resource_principal
to create appropriate authentication signer and kwargs required to instantiate theIdentityClient
object.serialize: (bool, optional)
. Defaults toTrue
. Whether to serialize the model to a pkl file by default. IfFalse
, you must set themodel_file_name
in.prepare
function, serialize the model manually, save it underartifact_dir
, and update thescore.py
file manually.
If you would like to serialize a model your way, you can set serialize=False
, and then save the serialized model into the artifact_dir
. However, you are also responsible for setting the model_file_name
in .prepare
function, making changes to load_model
and other functions in score.py
. Also, in score.py
, pre_inference
and post_inference
are currently not used.
properties
is an instance of ModelProperties
and has the following predefined fields:
inference_conda_env: str
inference_python_version: str
training_conda_env: str
training_python_version: str
training_resource_id: str
training_script_path: str
training_id: str
compartment_id: str
project_id: str
deployment_instance_shape: str
deployment_instance_count: int
deployment_bandwidth_mbps: int
deployment_log_group_id: str
deployment_access_log_id: str
deployment_predict_log_id: str
By default, properties
is populated from environment variables if it’s
not specified. For example, in the notebook session the environment variables
for project id and compartment id are preset and stored in PROJECT_OCID
and
NB_SESSION_COMPARTMENT_OCID``by default. And ``properties
populates these variables
from the environment variables, and uses the values in functions such as .save()
, .deploy()
by default.
However, if these aren’t the values you want, you can always explicitly pass the variables into functions to overwrite
those values. For the fields that properties
has, it records the values that you pass into the functions.
For example, when you pass inference_conda_env
into .prepare()
, then properties
records this value.
Later you can export it using .to_yaml()
, and reload it using .from_yaml()
from any machine.
This allows you to reuse the properties in different places.
Summary_status
You can call the summary_status()
function any time after the GenericModel
instance is created. This applies to other model frameworks such as SklearnModel
and so on. It returns a Pandas dataframe that guides you though the whole workflow. It shows which function is available to call, which ones aren’t and what each function is doing. If extra actions are required, it also shows those.
An example of a summary status table looks similar to the following after you initiate the model instance. The step column shows all the functions. It shows that init
step is completed where the Details
column explained that what the init
step did and now prepare()
is available. The next step is to call prepare()
.
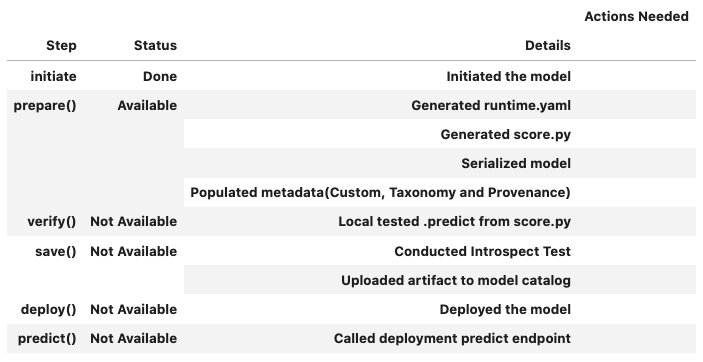
Prepare
.prepare()
takes the following parameters:
inference_conda_env: (str, optional)
. Defaults toNone
. Can be either a slug or an object storage path of the conda environment. You can only pass in slugs if the conda pack is a service environment.inference_python_version: (str, optional)
. Defaults toNone
. Python version to use in deployment.training_conda_env: (str, optional)
. Defaults toNone
. Can be either a slug or an object storage path of the conda environment. You can only pass in slugs if the conda environment is a service environment.training_python_version: (str, optional)
. Defaults toNone
. Python version used during training.model_file_name: (str)
. Name of the serialized model.as_onnx: (bool, optional)
. Defaults toFalse
. Whether to serialize as Onnx model. This will only enforce themodel_file_name
to have a suffix of.onnx
. The actually onnx serialization is not implemented here.force_overwrite: (bool, optional)
. Defaults toFalse
. Whether to overwrite existing files.namespace: (str, optional)
. Namespace of region. This is used for identifying which region the service environment is from when you pass a slug toinference_conda_env
andtraining_conda_env
.use_case_type: str
. The use case type of the model. Use it throughUserCaseType
class or string provided inUseCaseType
. For example,use_case_type=UseCaseType.BINARY_CLASSIFICATION
oruse_case_type="binary_classification"
. Check usingUseCaseType
class to see all of the supported types.X_sample: Union[list, pd.Series, np.ndarray, pd.DataFrame]
. Defaults toNone
. A sample of input data to use to generate input schema.y_sample: Union[list, pd.Series, np.ndarray, pd.DataFrame]
. Defaults toNone
. A sample of output data to use to generate output schema.training_script_path: str
. Defaults toNone
. Training script path.training_id: (str, optional)
. Defaults to the value from environment variables. The training OCID for model. Can be a notebook session or job OCID.ignore_pending_changes: bool
. Defaults toFalse
. Whether to ignore the pending changes in Git.max_col_num: (int, optional)
. Defaults toutils.DATA_SCHEMA_MAX_COL_NUM
. The maximum column size of the data that allows you to automatically a generate schema.
kwargs
:
impute_values: (dict, optional)
. The dictionary where the key is the column index (or names is accepted for pandas dataframe), and the value is the impute value for the corresponding column.
It automatically generates the following files.
runtime.yaml
score.py
.load_model
function is only implemented whenserialize=True
, otherwise, you need to implement it on your own.model.pkl
by default model is serialized in pkl format whenserialize=True
in theinit
function. Ifserialize=False
, the model isn’t automatically serialized.input_schema.json
whenX_sample
is passed in and the schema is more than 32 KB.output_schema.json
wheny_sample
is passed in and the schema is more than 32 KB.hyperparameters.json
if the extracted hyperparameters are more than 32KB.
Verify
The .verify()
function takes one parameter:
data (Union[dict, str, tuple, list])
. Data used to test if deployment works in a local environment.
You use it to test if deployment would work in the local environment. Before saving and deploying the model, we recommended that you call this function to check if the load_model
and predict
functions in score.py
work. It takes and returns the same data as model deployment predict takes and returns.
In GenericModel
data serialization is not supported, which means that you still must ensure that you pass in JSON serializable data to the verify
and predict
functions unless you implement extra data serialization and deserialization. Also, in the generated score.py
template,
Save
The Save
function takes the following parameters:
display_name: (str, optional)
. Defaults toNone
. The name of the model.description: (str, optional)
. Defaults toNone
. The description of the model.freeform_tags : Dict(str, str)
. Defaults toNone.
Free form tags for the model.defined_tags : (Dict(str, dict(str, object)), optional)
. Defaults toNone
. Defined tags for the model.ignore_introspection: (bool, optional)
. Defaults toNone
. Determines whether to ignore the result of model introspection or not. If set to True, the save ignores all model introspection errors.
kwargs
:
- project_id: (str, optional)
. Project OCID. If not specified, the value is taken either from the environment variables or model properties.
- compartment_id : (str, optional)
. Compartment OCID. If not specified, the value is taken either from the environment variables or model properties.
- timeout: (int, optional)
. Defaults to 10 seconds. The connection timeout in seconds for the client.
It first reloads the score.py
and runtime.yaml
files from disk so that any changes made to those files are used. Then it conducts an introspection test by default. However, you can set ignore_introspection=False
to avoid it. Introspection tests check if .deployment()
could have some issues and suggests necessary actions about how to fix them. Lastly, it uploads the artifacts to the model catalog, and returns a model_id
for the saved model.
You can also call .instrospect()
to conduct the test any time after you call .prepare()
.
Deploy
.deploy()
takes the following parameters:
wait_for_completion : (bool, optional)
. Defaults toTrue
. Set to wait for the deployment to complete before proceeding.display_name: (str, optional)
. Defaults toNone
. The name of the model.description: (str, optional)
. Defaults toNone
. The description of the model.deployment_instance_shape: (str, optional)
. Default toVM.Standard2.1
. The shape of the instance used for deployment.deployment_instance_count: (int, optional)
. Defaults to 1. The number of instances used for deployment.deployment_bandwidth_mbps: (int, optional)
. Defaults to 10. The bandwidth limit on the load balancer in Mbps.deployment_log_group_id: (str, optional)
. Defaults toNone
. The oci logging group id. The access log and predict log share the same log group.deployment_access_log_id: (str, optional)
. Defaults toNone
. The access log OCID for the access logs, see linkdeployment_predict_log_id: (str, optional)
. Defaults toNone
. The predict log OCID for the predict logs, see link
kwargs
:
- project_id: (str, optional)
. Project OCID. If not specified, the value is taken from the environment variables.
- compartment_id : (str, optional)
. Compartment OCID. If not specified, the value is taken from the environment variables.
- max_wait_time : (int, optional)
. Defaults to 1200 seconds. Maximum amount of time to wait in seconds. Negative implies an infinite wait time.
- poll_interval : (int, optional)
. Defaults to 60 seconds. Poll interval in seconds.
It deploys the model. In order to make deployment more smooth, we suggest using exactly the same conda environments for both local development and deployment. Discrepancy between the two could cause problems.
You can pass in deployment_log_group_id
, deployment_access_log_id
and deployment_predict_log_id
to enable the logging. To create a log group, you can reference Logging.
Logging Example
import tempfile
from ads.model.generic_model import GenericModel
log_group_ocid = 'ocid1.loggroup.oc1.iad.amaaaaaav...'
log_ocid = 'ocid1.log.oc1.iad.amaaaaaav66vvniagrvj...'
class Toy:
def predict(self, x):
return x ** 2
estimator = Toy()
model = GenericModel(estimator=estimator, artifact_dir=tempfile.mkdtemp())
model.summary_status()
model.prepare(inference_conda_env="dataexpl_p37_cpu_v3")
model.verify(2)
model.save()
model.deploy(
deployment_log_group_id=log_group_ocid,
deployment_access_log_id=log_ocid,
deployment_predict_log_id =log_ocid,
)
model.predict(2)
model.model_deployment.show_logs(log_type="predict")
model.model_deployment.show_logs(log_type="access")
model.model_deployment.access_log.tail()
model.model_deployment.predict_log.tail()
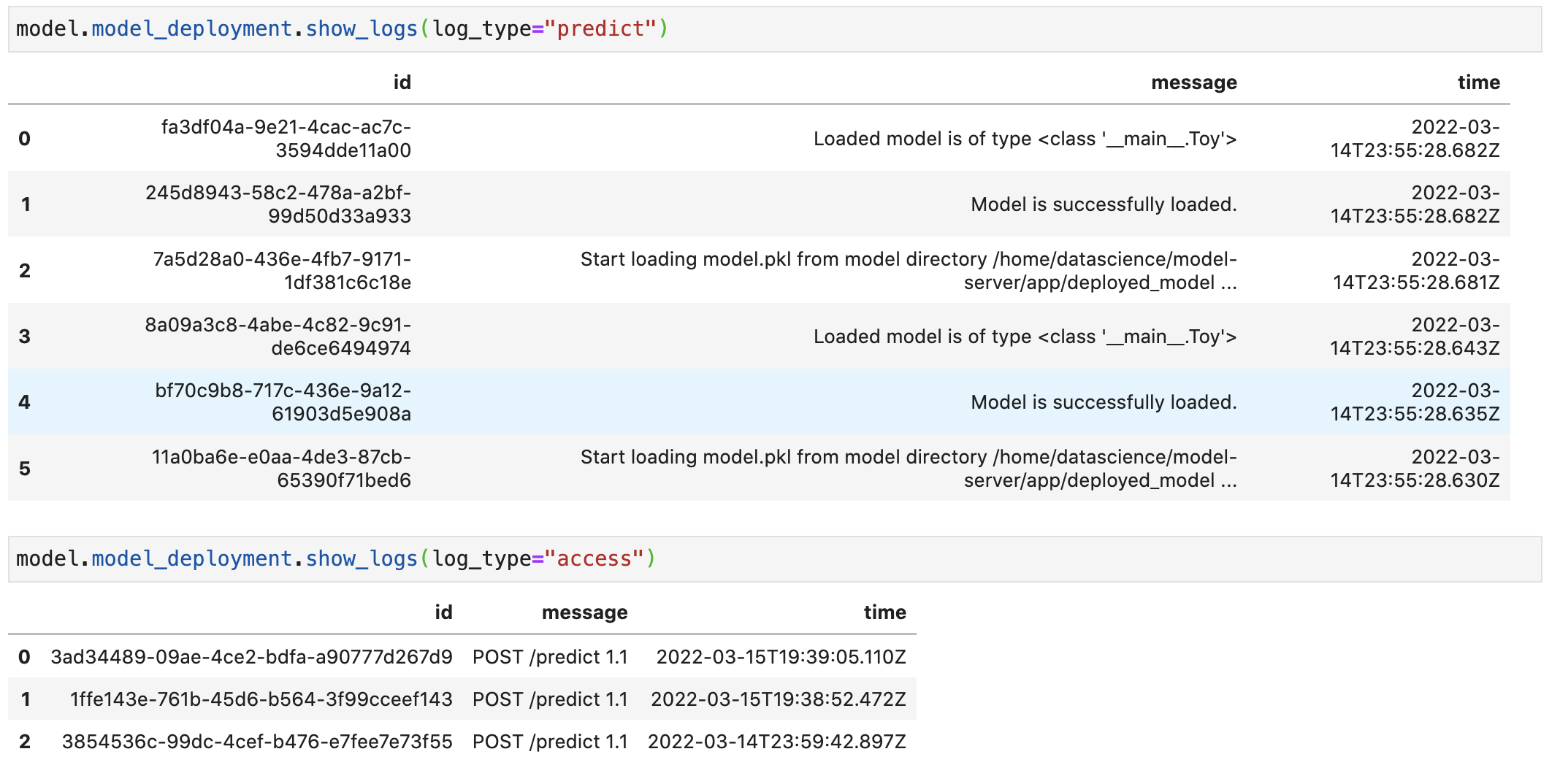
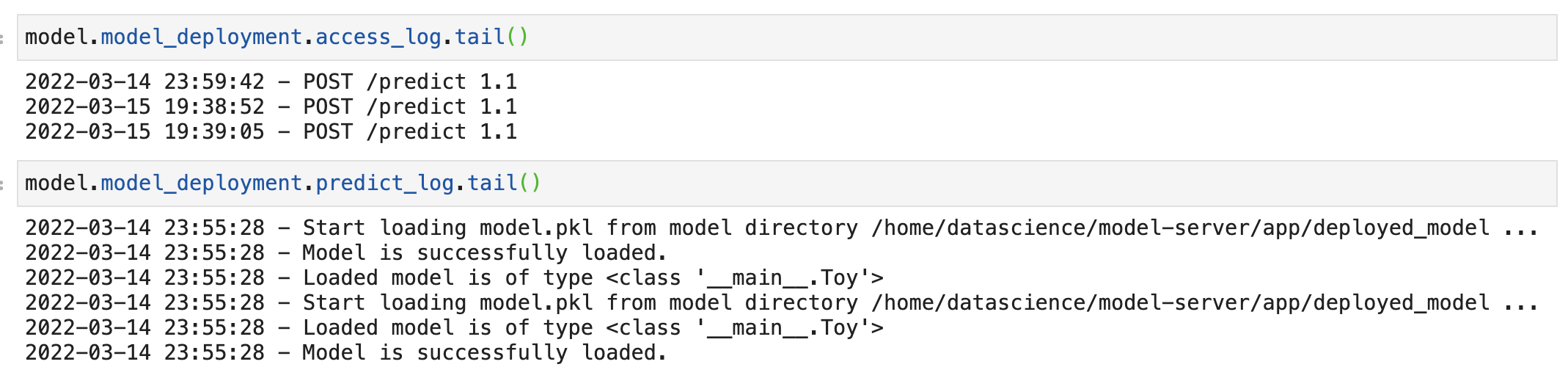
Predict
.predict()
takes one parameter:
- data: Union[dict, str, tuple, list]
. JSON serializable data for the prediction for Onnx models. For the local serialization method, the data can be the data types that each framework support.
.predict()``takes the same data that ``.verify()
takes. You must ensure that the data passed and returned by predict
in the score.py
is JSON serializable. It passes the data to the model deployment endpoint and calls the predict
function in score.py
.
Delete_deployment
.delete_deployment()
takes one parameter:
wait_for_completion: (bool, optional)
. Defaults to False. Whether to wait till completion.
When you don’t need the deployment any more, call delete_deployment
to delete the current deployment that is attached to the model. Note that each time you call deploy, it creates a new deployment and only the new deployment is attached to the model.
from_model_artifact
.from_model_artifact()
allows to load a model from a folder, zip or tar achive files, where the folder/zip/tar files should contain the files such as runtime.yaml, score.py, the serialized model file needed for deployments. It takes the following parameters:
uri: str
: The folder path, ZIP file path, or TAR file path. It could contain a seriliazed model(required) as well as any files needed for deployment including: serialized model, runtime.yaml, score.py and etc. The content of the folder will be copied to theartifact_dir
folder.model_file_name: str
: The serialized model file name.artifact_dir: str
: The artifact directory to store the files needed for deployment.auth: (Dict, optional)
: Defaults to None. The default authetication is set usingads.set_auth
API. If you need to override the default, use the ads.common.auth.api_keys or ads.common.auth.resource_principal to create appropriate authentication signer and kwargs required to instantiate IdentityClient object.force_overwrite: (bool, optional)
: Defaults to False. Whether to overwrite existing files or not.properties: (ModelProperties, optional)
: Defaults to None. ModelProperties object required to save and deploy model.
After this is called, you can call .verify()
, .save()
and etc.
from_model_catalog
from_model_catalog
allows to load a remote model from model catalog using a model id , which should contain the files such as runtime.yaml, score.py, the serialized model file needed for deployments. It takes the following parameters:
model_id: str
. The model OCID.model_file_name: (str)
. The name of the serialized model.artifact_dir: str
. The artifact directory to store the files needed for deployment. Will be created if not exists.auth: (Dict, optional)
. Defaults to None. The default authetication is set usingads.set_auth
API. If you need to override the default, use theads.common.auth.api_keys
orads.common.auth.resource_principal
to create appropriate authentication signer and kwargs required to instantiate IdentityClient object.force_overwrite: (bool, optional)
. Defaults to False. Whether to overwrite existing files or not.properties: (ModelProperties, optional)
. Defaults to None. ModelProperties object required to save and deploy model.
kwargs
:
compartment_id : (str, optional)
. Compartment OCID. If not specified, the value will be taken from the environment variables.timeout : (int, optional)
. Defaults to 10 seconds. The connection timeout in seconds for the client.
Examples
Serialize the Model to pkl Format by Default
import tempfile
from ads.model.generic_model import GenericModel
class Toy:
def predict(self, x):
return x ** 2
estimator = Toy()
model = GenericModel(estimator=estimator, artifact_dir=tempfile.mkdtemp())
model.summary_status()
model.prepare(inference_conda_env="oci://service-conda-packs@id19sfcrra6z/service_pack/cpu/Data Exploration and Manipulation for CPU Python 3.7/3.0/dataexpl_p37_cpu_v3",
inference_python_version="3.7",
model_file_name="toy_model.pkl",
training_id=None,
force_overwrite=True
)
model.verify(2)
model.save()
model.deploy()
model.predict(2)
model.delete_deployment()
Serialize the Model In Your Way
import tempfile
from ads.model.generic_model import GenericModel
class Toy:
def predict(self, x):
return x ** 2
estimator = Toy()
model = GenericModel(estimator=estimator,
artifact_dir=tempfile.mkdtemp(),
serialize=False)
model.summary_status()
model.prepare(inference_conda_env="oci://service-conda-packs@id19sfcrra6z/service_pack/cpu/Data Exploration and Manipulation for CPU Python 3.7/3.0/dataexpl_p37_cpu_v3",
inference_python_version="3.7",
model_file_name="toy_model.pkl",
training_id=None,
force_overwrite=True
)
model.summary_status()
# Serialize the model
import cloudpickle
import os
with open(os.path.join(model.artifact_dir, "toy_model.pkl"), "wb") as outfile:
cloudpickle.dump(estimator, outfile)
# Finish implementing the score.py
score = '''
import json
import os
from cloudpickle import cloudpickle
model_name = 'toy_model.pkl'
def load_model(model_file_name=model_name):
"""
Loads model from the serialized format
Returns
-------
model: a model instance on which predict API can be invoked
"""
model_dir = os.path.dirname(os.path.realpath(__file__))
contents = os.listdir(model_dir)
if model_file_name in contents:
with open(os.path.join(os.path.dirname(os.path.realpath(__file__)), model_file_name), "rb") as file:
return cloudpickle.load(file)
else:
raise Exception('{0} is not found in model directory {1}'.format(model_file_name, model_dir))
def predict(data, model=load_model()):
"""
Returns prediction given the model and data to predict
Parameters
----------
model: Model instance returned by load_model API
data: Data format as expected by the predict API of the core estimator. For eg. in case of sckit models it could be numpy array/List of list/Panda DataFrame
Returns
-------
predictions: Output from scoring server
Format: {'prediction':output from model.predict method}
"""
return {'prediction':model.predict(data)}
'''
with open(os.path.join(model.artifact_dir, "score.py"), 'w') as f:
print(f.write(score))
model.verify(2)
model.save()
model.deploy(deployment_log_group_id="ocid1.loggroup.oc1.iad.amaaaa...",
deployment_access_log_id="ocid1.log.oc1.iad.amaaaaaav...",
deployment_predict_log_id="ocid1.log.oc1.iad.amaaaaaav...")
model.delete_deployment()
Loading Model From a Zip Archive
model = GenericModel.from_model_artifact("/folder_to_your/artifact.zip",
model_file_name="your_model_file_name",
artifact_dir=tempfile.mkdtemp())
model.verify(your_data)
Loading Model From Model Catalog
model = GenericModel.from_model_catalog(model_id="ocid1.datasciencemodel.oc1.iad.amaaaa....",
model_file_name="your_model_file_name",
artifact_dir=tempfile.mkdtemp())
model.verify(your_data)